Team up with open-source AI for seamless screening
Performance boost
Human in the loop
You are the expert, the machine assists you.
Cutting-edge research
Built-on and improved by ongoing research.
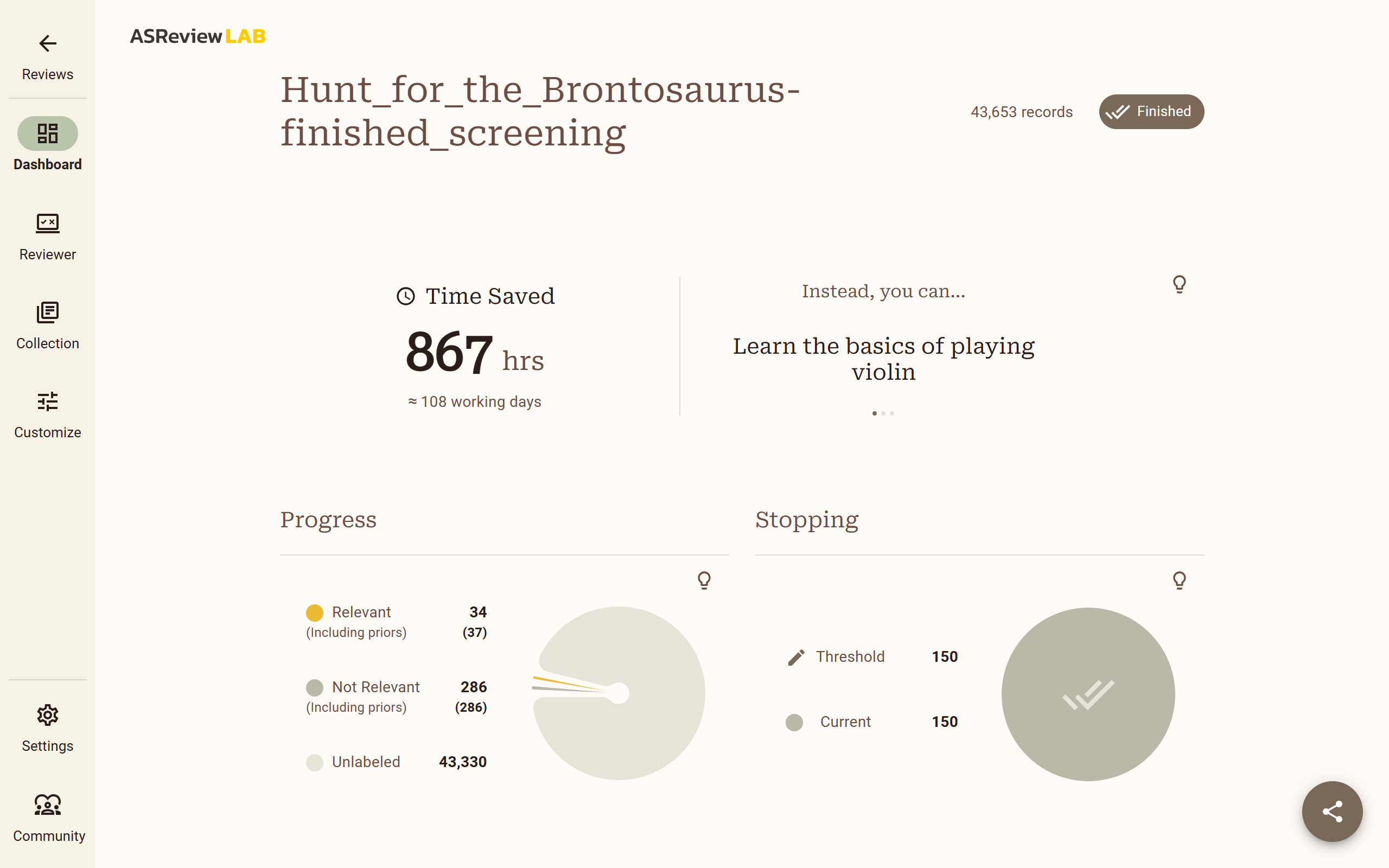
Smarter by default
The best choice is already made
No need to choose. The new default model is faster, smarter, and ready to go.
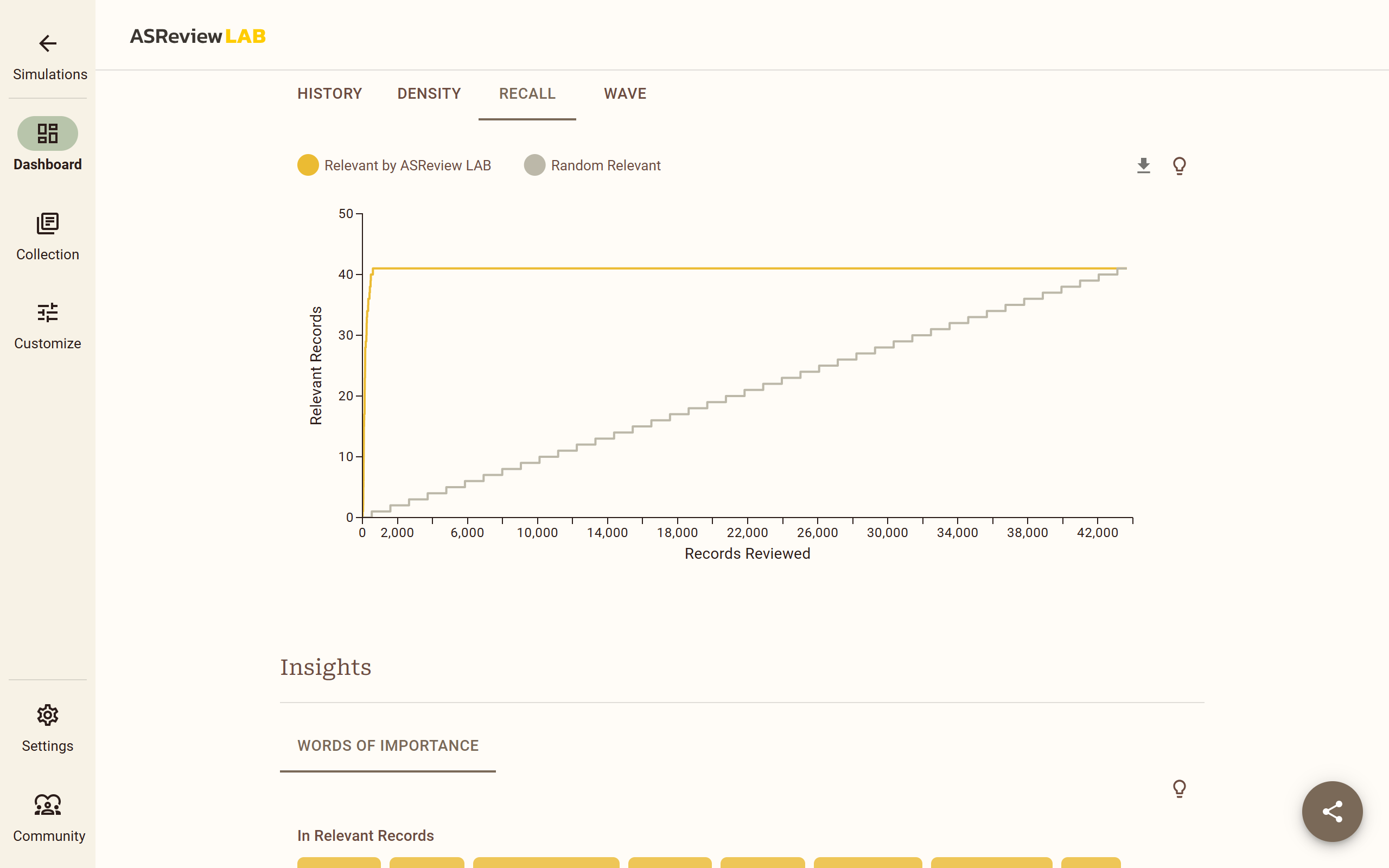
Hot start
Use your prior knowledge to give the model a boost.
Context-aware
Understands meaning, not just words without any extra installs like Dory needed.
“Throughout the research world, artificial intelligence is increasingly being applied to scanning complicated scientific literature more quickly than humans alone can do. ”
Science Business
Light-weight or Super Heavy AI
From good to super advanced AI
Adaptable for every kind of user, offering the flexibility to utilize either lightweight or heavyweight AI models based on specific needs.
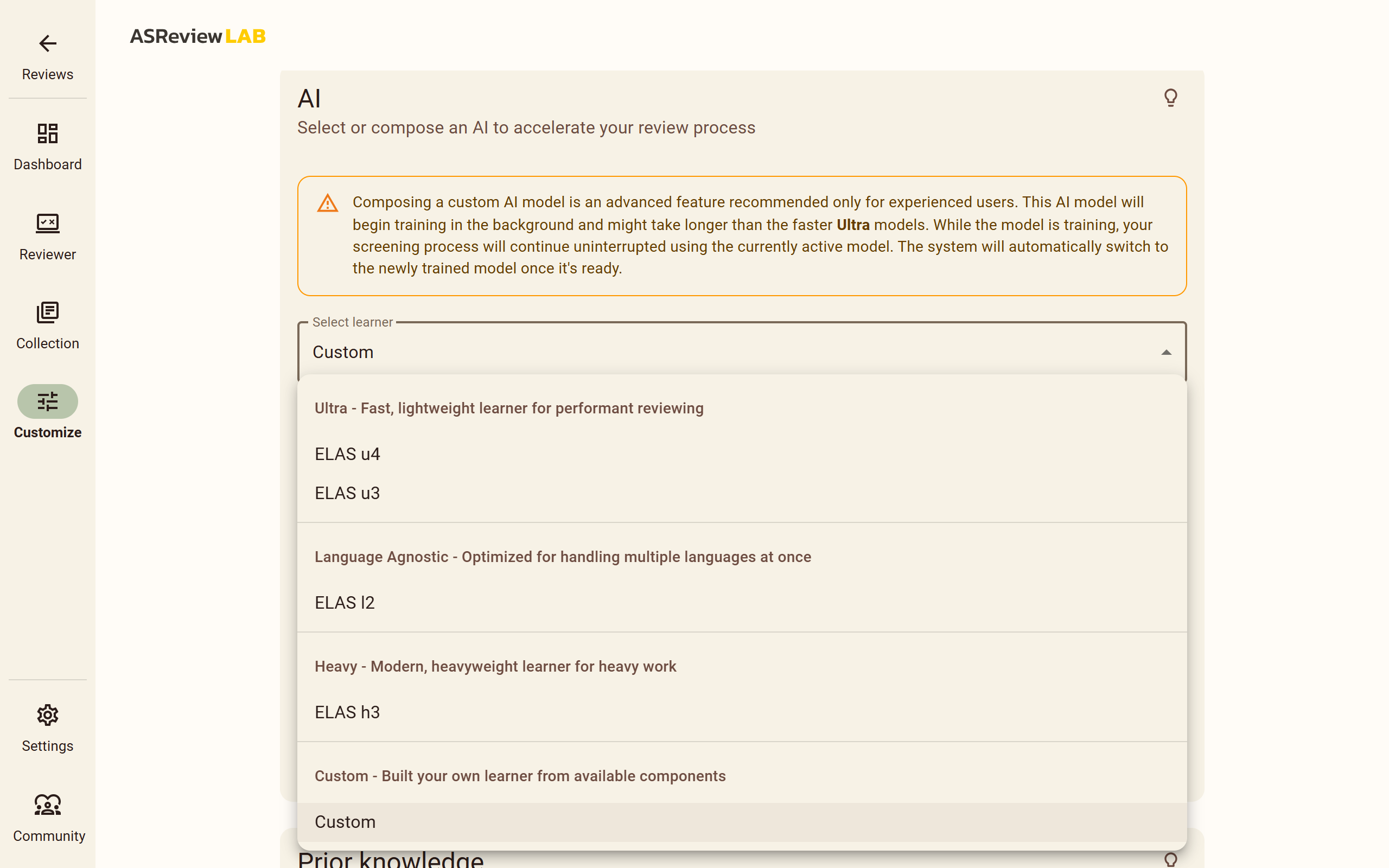
Screen interactively with AI
Unlock real-time learning with AI
Harness the power of adaptive algorithms which become smarter after each labeling decision.
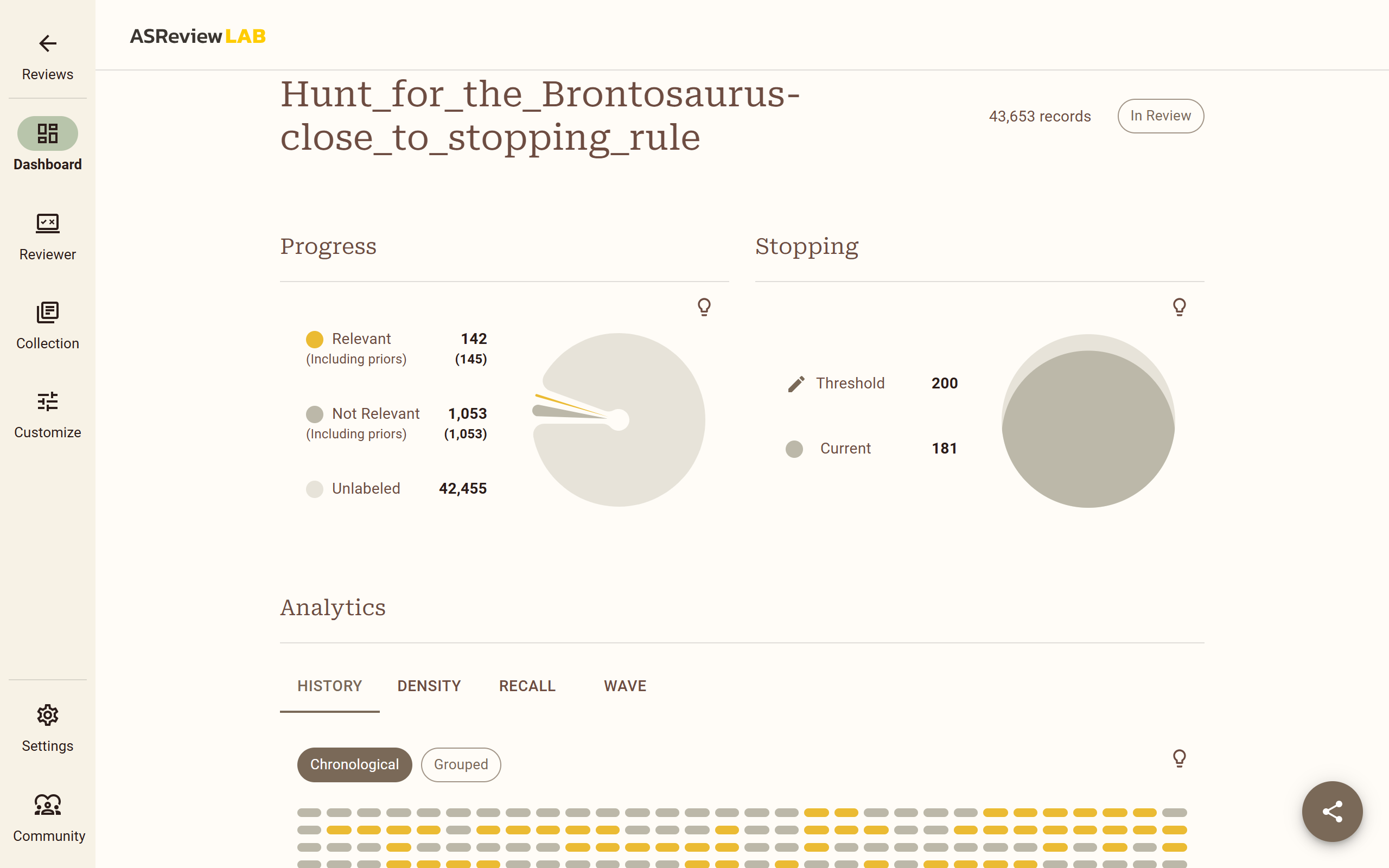
Most relevant texts
Receive the most relevant texts based on the scope of your search.
Personalized learning
Create customized pathways with AI based on your learning style.
Immediate feedback
Give instant feedback to help the AI understand your needs.
Open AI you can trust
Fully reproducible and transparent AI
Every decision, whether human or AI, is made crystal clear and fully repeatable.
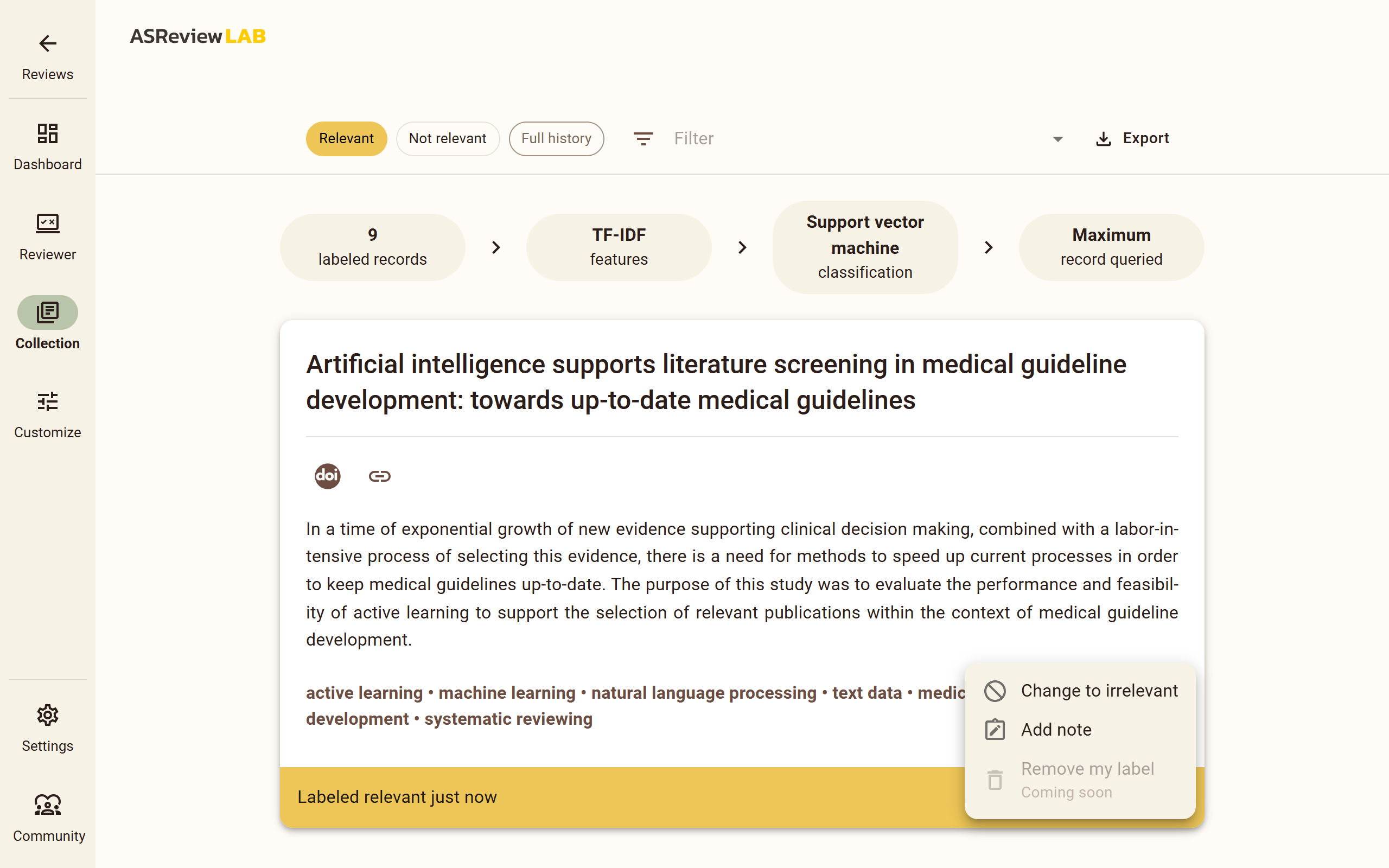
Open models
Only open-source models are used, no black box involved.
Transparancy
Reproducible
All steps are retractable complying to the highest level of compliance.
Experience ASReview AI
Find out how our AI helps you with a 95% disappearing act.
Questions & Answers
How does ASReview balance automation with human decision-making in the review process?
ASReview uses active learning to focus on the most relevant records while keeping a human in the loop as the final decision-maker (the “oracle”). Unlike fully automated approaches (e.g., using large language models, which can introduce false outputs or “hallucinations”), active learning pairs the strengths of AI with human expertise. This helps minimize errors that arise from overly narrow search filters or fully automated screening. For more insights on this approach, see this paper.
What are the benefits of using AI-driven active learning compared to traditional screening methods?
AI-driven active learning can substantially reduce the time spent reviewing irrelevant records, allowing researchers to identify key studies more quickly and reliably. This method has been shown to outperform manual screening in simulation studies mimicking the screening process. For a deeper exploration of these benefits, read this paper.
How does ASReview ensure transparency, interpretability, and reliability in its AI models?
ASReview emphasizes open-source development, clear documentation of each screening step, and detailed logging of user decisions and AI results. For further discussion on these practices, consult this paper.
How does ASReview use active learning to improve the systematic review process?
ASReview’s active learning engine prioritizes records most likely to be relevant, presenting them to the human screener for confirmation. Each decision refines the AI’s understanding of what you’re seeking, leading to faster and more accurate retrieval of the target studies. This approach, described as “researcher-in-the-loop” or Active Learning To Rank, has shown strong performance in simulation studies. More details can be found here.
What types of AI models are used in ASReview, and what does the numbers mean (e.g., u3 versus u4)?
ASReview offers three sets of AI models:
The ELAS-Ultra series is always available and balances speed with accuracy, while taking some context into account.
The ELAS-Heavy series uses semantic models, useful when you have enough labeled data and want to take even more context into account.
The ELAS-L series is language-agnostic and suited for multi-language reviews.
The latter two require the Dory package.
Each AI model can have multiple versions, indicated by a number after the model. The highest number refers to the specific set’s latest and best version of the AI model.
Subscribe to our newsletter!
Stay on top of ASReview’s developments by subscribing to the newsletter.